AI in Healthcare:
Artificial intelligence (AI) is the use of computers and machine processes to simulate human intelligence and perform complex automated tasks. AI has already changed many aspects of our lives, from automating systems to improving the decisions we make and the ways we go about making them.
However, one of the most impactful and personal ways AI is changing our world is within the field of healthcare, where it is being used to enhance a variety of common medical processes, such as diagnosing diseases, recommending treatments, guiding surgical care, monitoring patients, and supporting population health management.
In this article, you will learn more about the types of AI used in healthcare, some of their applications and the benefits of AI within the field, as well as the challenges, possible solutions, and future prospects of AI in healthcare. You will also explore relevant jobs and online courses that can help you get started using AI for healthcare purposes today.
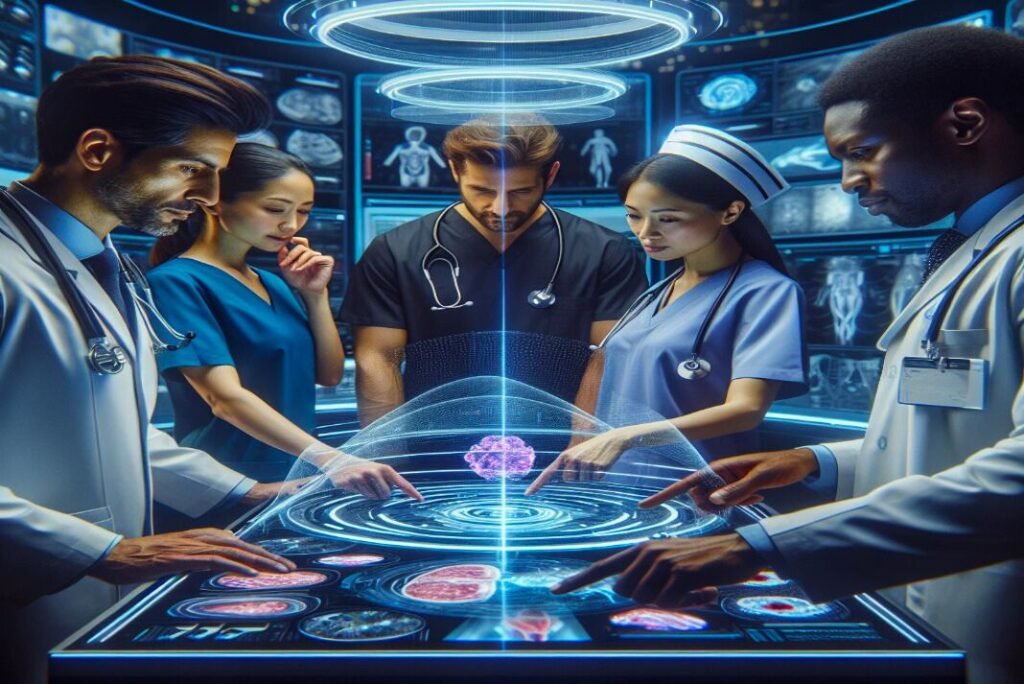
Types of AI in Healthcare
Artificial Intelligence is a general term that encompasses many different but related processes. Some of the most common forms of AI used within healthcare include:
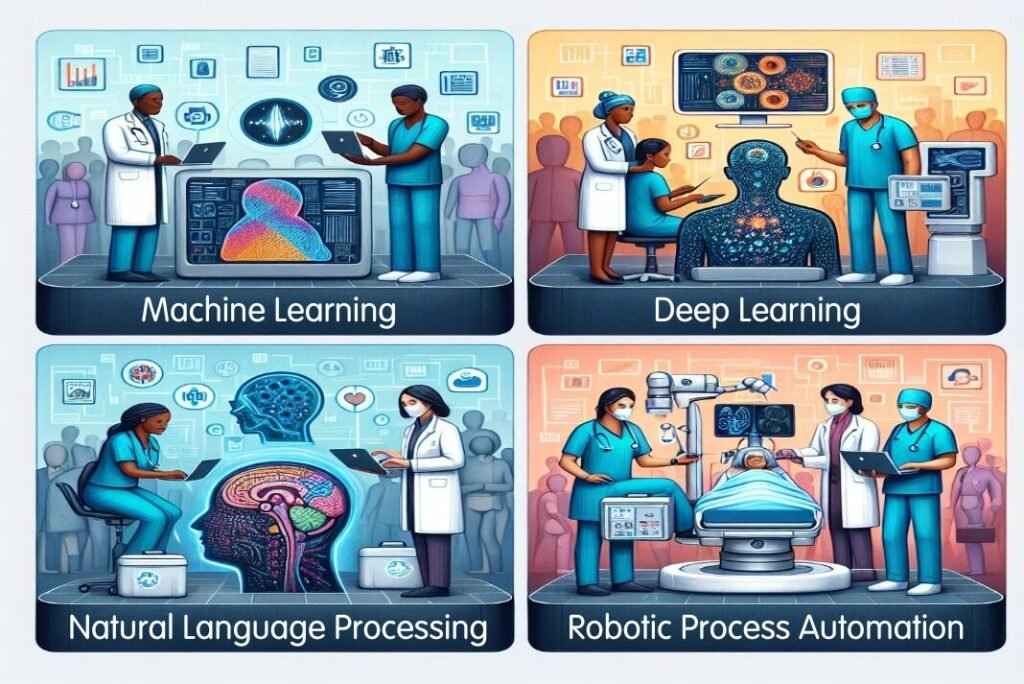
Machine Learning
Machine learning (ML) is the process of training algorithms using data sets, such as health records, to create models capable of performing such tasks as categorizing information or predicting outcomes. For example, ML can be used to analyze medical images, such as X-rays or MRI scans, and detect abnormalities or diagnose conditions.
Deep Learning
Deep learning is a subset of machine learning that involves greater volumes of data, training times, and layers of ML algorithms to produce neural networks capable of more complex tasks. For example, deep learning can be used to generate synthetic data, such as synthetic medical images, that can augment existing data and improve the performance of ML models.
Natural Language Processing
Natural language processing (NLP) is the use of ML to understand human language, whether it be verbal or written. In healthcare, NLP can be used to interpret documentation, notes, reports, and published research. For example, NLP can be used to extract relevant information from electronic health records (EHRs), such as patient symptoms, medications, or lab results, and use them to support clinical decision making.
Robotic Process Automation
Robotic process automation (RPA) is the use of AI in computer programs to automate administrative and clinical workflows. These programs can mimic human actions, such as clicking, typing, or copying, and perform them faster and more accurately. For example, RPA can be used to automate tasks such as scheduling appointments, billing, or claims processing, and reduce the burden on healthcare staff.
Applications of AI in Healthcare
As artificial intelligence becomes more widely adopted, so too does the number of ways the technology is being used across the health sector. Some of the most prominent applications of AI in healthcare include:
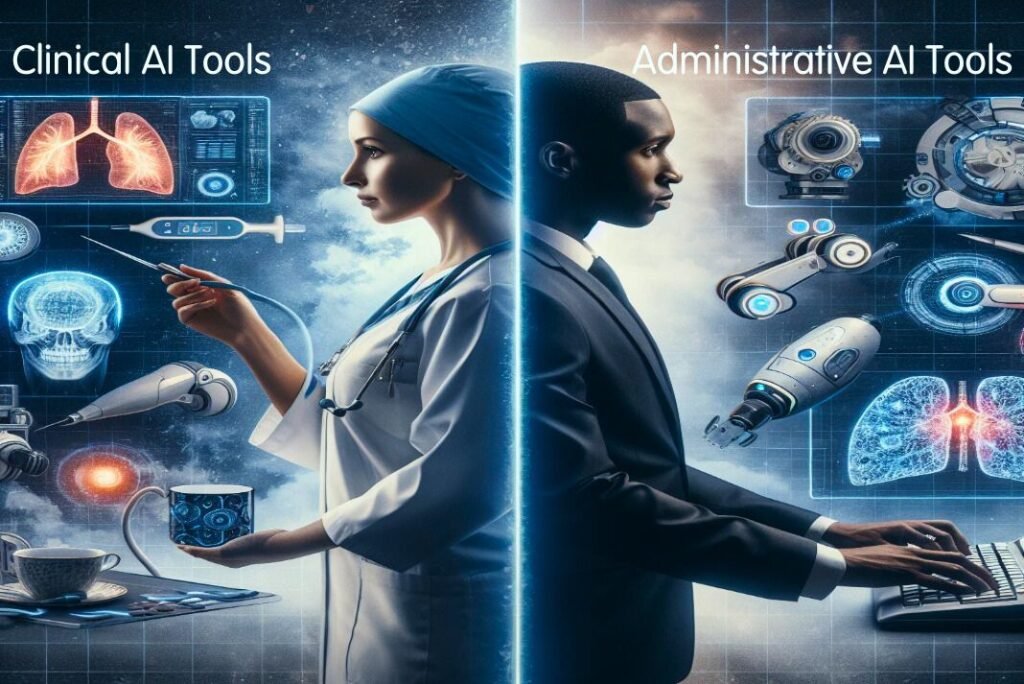
Clinical AI Tools
Clinical AI tools are those that directly assist with patient care, such as:
- Predicting health trajectories of patients, such as their risk of developing certain diseases, their response to treatments, or their likelihood of survival.
- Recommending treatments, such as drugs, dosages, or therapies, based on the patient’s condition, preferences, and medical history.
- Guiding surgical care, such as using robotic surgical equipment outfitted with AI to help surgeons better perform surgeries by decreasing their physical fluctuations and providing updated information during the operation.
- Monitoring patients, such as using wearable devices, sensors, or apps to track the patient’s vital signs, symptoms, or behaviors, and alert the healthcare providers or the patients themselves of any changes or anomalies.
- Supporting population health management, such as using AI to analyze large-scale data from various sources, such as EHRs, social media, or environmental factors, and identify patterns, trends, or risks that affect the health outcomes of a community.
Administrative AI Tools
Administrative AI tools are those that indirectly assist with patient care, such as:
- Recording digital notes, such as using speech recognition or NLP to transcribe the verbal interactions between the healthcare providers and the patients, and automatically document them in the EHRs.
- Optimizing operational processes, such as using AI to analyze data from various sources, such as staff schedules, patient flow, or inventory, and provide insights or suggestions to improve the efficiency and quality of the healthcare services.
- Automating laborious tasks, such as using RPA to perform repetitive or mundane tasks, such as data entry, verification, or extraction, and free up the time and resources of the healthcare staff.
Benefits of AI in Healthcare
AI has the potential to bring significant benefits to the healthcare sector, such as:
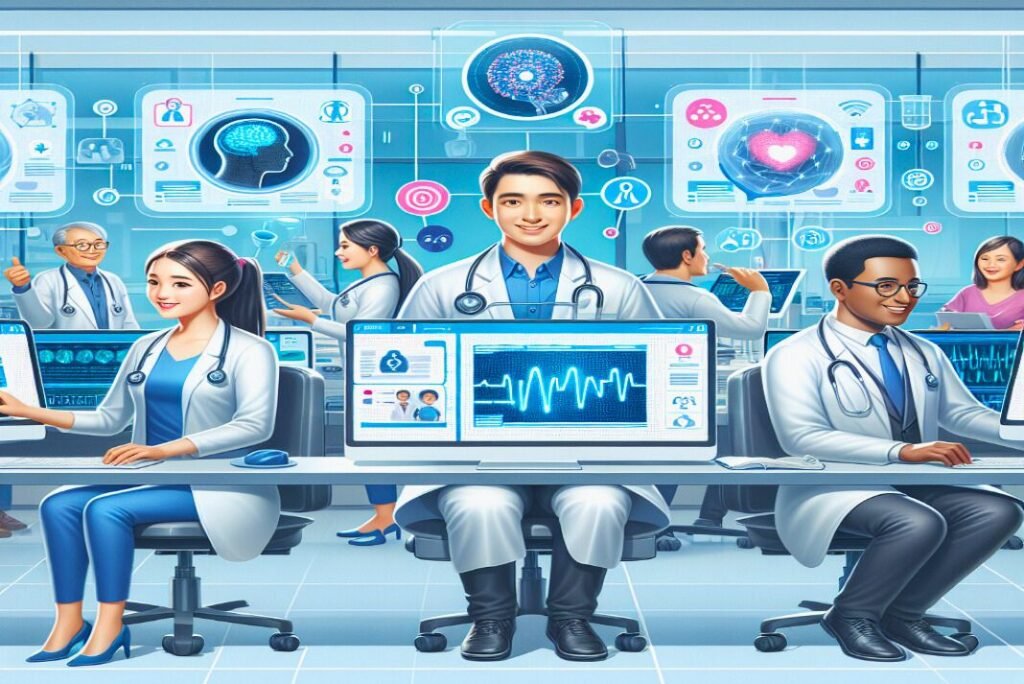
Improved Quality and Efficiency of Care
AI can help improve the quality and efficiency of care by:
- Providing faster and more accurate diagnoses, such as using ML to analyze medical images and detect diseases or conditions that may be missed by human eyes.
- Enhancing clinical decision support, such as using AI to provide relevant information, recommendations, or feedback to the healthcare providers, and help them make better and more informed decisions.
- Facilitating personalized and precision medicine, such as using AI to tailor treatments to the individual characteristics and needs of each patient, and optimize their effectiveness and safety.
- Enabling remote and virtual care, such as using AI to monitor patients at home, provide telehealth services, or deliver care through chatbots or avatars.
Reduced Costs and Errors
AI can help reduce costs and errors by:
- Automating tasks and processes, such as using RPA to perform administrative or clinical tasks that are time-consuming, tedious, or prone to human errors, and save money and manpower.
- Optimizing resource allocation, such as using AI to analyze data and provide insights or suggestions to improve the utilization and management of resources, such as staff, equipment, or supplies.
- Preventing adverse events, such as using AI to predict and prevent potential complications, errors, or harms, such as medication errors, hospital-acquired infections, or readmissions.
Enhanced Patient Experience and Outcomes
AI can help enhance patient experience and outcomes by:
- Improving patient engagement and empowerment, such as using AI to provide patients with information, education, or guidance, and help them manage their own health and wellness.
- Increasing patient satisfaction and loyalty, such as using AI to provide patients with personalized, convenient, and accessible care, and improve their communication and relationship with the healthcare providers.
- Improving patient quality of life and survival, such as using AI to provide patients with effective and safe treatments, prevent or delay the progression of diseases, or improve their functional abilities and well-being.
Increased Access and Equity
AI can help increase access and equity by:
- Expanding the reach and coverage of healthcare, such as using AI to provide healthcare services to underserved or remote populations, or to address the shortage or uneven distribution of healthcare workers.
- Reducing health disparities and inequalities, such as using AI to identify and address the social determinants of health, or the factors that influence the health status and outcomes of different groups of people, such as income, education, or race.
Challenges of AI in Healthcare and Possible Solutions
Despite the potential benefits, AI also faces several challenges that may impede its widespread adoption and impact in the healthcare sector, such as:
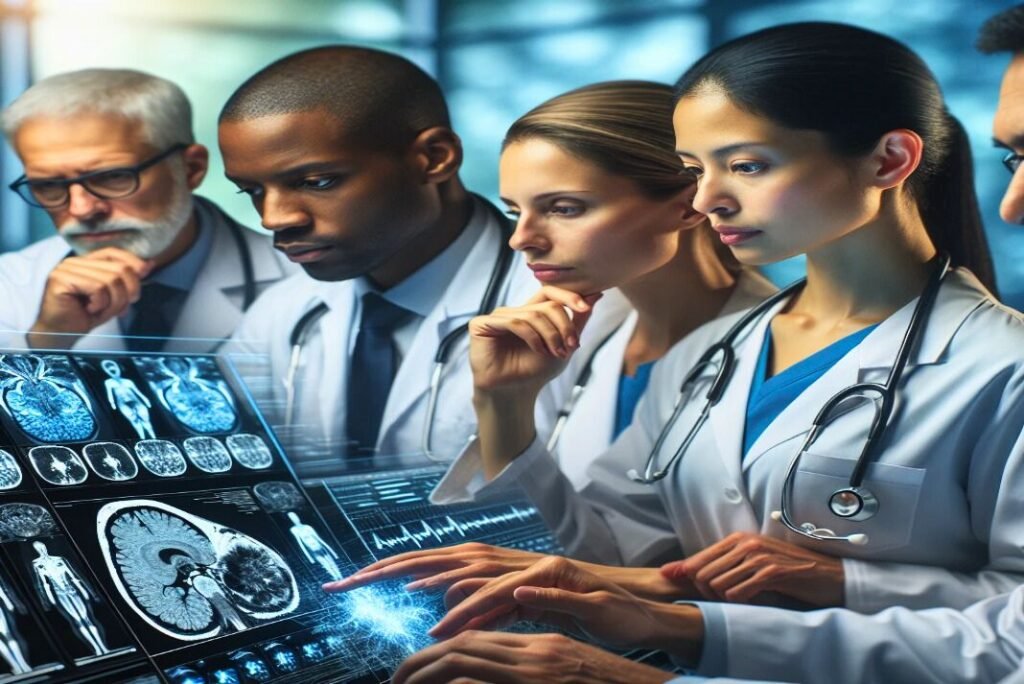
Data Access and Quality
AI tools need reliable, accurate, and representative data to perform well in healthcare. Data access and quality are the issues of making sure that the AI tools and their data sources and inputs are trustworthy and suitable for the healthcare domain and the target population. Data access and quality can be influenced by many factors, such as:
- Data availability and accessibility, such as how much, relevant, and timely data is there, and how easy or hard is it to access or share the data for the AI tools and their users.
- Data diversity and representativeness, such as how well the data reflects the diversity and characteristics of the population, and how fair or biased the data is for the AI tools and their outcomes or actions.
- Data integrity and security, such as how safe and secure the data is from corruption, tampering, or compromise, and how private and confidential the data is for the AI tools and their data sources and inputs.
Data access and quality can have significant impacts on the healthcare sector, such as:
- Affecting the accuracy and reliability of the AI results or actions, such as the quality and quantity of data can cause errors, mistakes, or harms in the AI outputs or decisions, or provide inaccurate, incomplete, or misleading information or recommendations to the healthcare providers or the patients.
- Affecting the equity and inclusivity of the healthcare services, such as the diversity and representativeness of data can create or worsen disparities, discrimination, or marginalization of some groups or subgroups of the population, or violate the rights and dignity of the patients.
- Affecting the compliance and accountability of the AI tools and the healthcare providers, such as the integrity and security of data can expose the AI tools and the healthcare providers to potential lawsuits, penalties, or sanctions, or violate the laws and regulations governing the healthcare sector.
To address the issues of data access and quality, some of the possible solutions include:
- Improving the data collection and generation, such as using more, relevant, or timely data, and making sure that the data sources and inputs are sufficient, reliable, and representative of the healthcare domain and the target population.
- Improving the data analysis and processing, such as using techniques to reduce, balance, or augment the data, and making sure that the data diversity and representativeness are preserved, enhanced, or accounted for in the AI tools and their results or actions.
- Improving the data protection and governance, such as using encryption, anonymization, or pseudonymization techniques, and making sure that the data integrity and security are maintained, monitored, and audited in the AI tools and their data sources and inputs.
Bias and Fairness
Bias and fairness are the issues of ensuring that AI tools do not produce or perpetuate unfair or discriminatory outcomes for certain groups of people, such as based on their gender, race, or age. Bias and fairness can be affected by various factors, such as:
- Data bias, such as the lack of representation, diversity, or balance in the data used to train or test the AI tools, which may lead to skewed or inaccurate results.
- Algorithm bias, such as the use of inappropriate, flawed, or hidden assumptions, rules, or criteria in the AI tools, which may lead to biased or unjust decisions.
- Human bias, such as the influence of conscious or unconscious prejudices, stereotypes, or expectations of the human users, developers, or regulators of the AI tools, which may lead to biased or unethical behaviors.
Bias and fairness can affect healthcare in many ways, such as:
- Affecting the quality and safety of care, such as by misdiagnosing, mistreating, or harming certain patients, or by providing ineffective or inappropriate treatments or recommendations.
- Affecting the trust and confidence of the patients and the public, such as by undermining the credibility, accountability, and transparency of the AI tools and the healthcare providers, or by violating the rights and dignity of the patients.
- Affecting the legal and ethical implications, such as by exposing the AI tools and the healthcare providers to potential lawsuits, penalties, or sanctions, or by violating the rules of healthcare.
Some of the possible solutions for bias and fairness are:
- Improving the data quality and diversity, such as by collecting, cleaning, and labeling more and better data, and making sure that the data reflects the characteristics and needs of the target population.
- Improving the algorithm design and evaluation, such as by using appropriate, robust, and transparent methods, metrics, and standards, and testing and validating the AI tools against various scenarios and benchmarks.
- Improving the human oversight and involvement, such as by educating, training, and empowering the human users, developers, and regulators of the AI tools, and making sure that they have the ability and responsibility to monitor, review, and intervene in the AI processes and outcomes.
Scaling and Integration
Scaling and integration are the challenges of deploying and implementing the AI tools in the real-world healthcare settings, and ensuring that they work well with the existing systems, processes, and people. Scaling and integration can be hindered by various factors, such as:
- Technical barriers, such as the lack of compatibility, interoperability, or standardization among different AI tools, platforms, or devices, or the lack of infrastructure, bandwidth, or security for the AI operations.
- Organizational barriers, such as the lack of alignment, coordination, or collaboration among different stakeholders, such as the healthcare providers, administrators, or regulators, or the lack of policies, guidelines, or incentives for the AI adoption and integration.
- Cultural barriers, such as the lack of awareness, understanding, or acceptance of the AI tools among the healthcare staff, patients, or public, or the lack of trust, confidence, or satisfaction with the AI tools and their impact on the healthcare delivery and outcomes.
Scaling and integration can affect healthcare in many ways, such as:
- Affecting the performance and reliability of the AI tools, such as by causing errors, failures, or inconsistencies in the AI results or actions, or by compromising the quality and safety of the AI tools and the healthcare services.
- Affecting the efficiency and effectiveness of the healthcare processes, such as by creating bottlenecks, redundancies, or conflicts in the healthcare workflows, or by wasting time, money, or resources in the AI deployment and maintenance.
- Affecting the innovation and competitiveness of the healthcare sector, such as by limiting the potential, scope, or impact of the AI tools and their applications, or by losing the opportunity, advantage, or edge in the AI development and market.
Some of the possible solutions for scaling and integration are:
- Improving the technical compatibility and interoperability, such as by using common, open, or flexible standards, protocols, or interfaces, and making sure that the AI tools can communicate, exchange, or integrate data and information with other systems, platforms, or devices.
- Improving the organizational alignment and coordination, such as by establishing clear, consistent, and comprehensive policies, guidelines, or incentives, and making sure that the AI tools are aligned with the goals, values, and needs of the healthcare sector and its stakeholders.
- Improving the cultural awareness and acceptance, such as by educating, informing, or engaging the healthcare staff, patients, or public, and making sure that the AI tools are user-friendly, accessible, and beneficial for the healthcare sector and its beneficiaries.
Transparency and Explainability
Transparency and explainability are the issues of ensuring that the AI tools and their processes and outcomes are understandable, interpretable, and accountable to the human users, developers, and regulators. Transparency and explainability can be affected by various factors, such as:
- Data complexity and opacity, such as the use of large, heterogeneous, or dynamic data sets, or the lack of data documentation, annotation, or provenance, which may make it hard to trace or verify the data sources, quality, or usage.
- Algorithm complexity and opacity, such as the use of sophisticated, nonlinear, or adaptive algorithms, or the lack of algorithm documentation, validation, or evaluation, which may make it hard to understand or predict the logic, rationale, or criteria of the AI tools and their results.
- Human complexity and opacity, such as the use of subjective, implicit, or contextual factors, or the lack of human feedback, communication, or interaction with the AI tools, which may make it hard to comprehend or justify the expectations, preferences, or behaviors of the human actors in the AI processes and outcomes.
Transparency and explainability can affect healthcare in many ways, such as:
- Affecting the quality and safety of care, such as by causing errors, mistakes, or harms in the AI results or actions, or by giving wrong, incomplete, or misleading information or recommendations to the healthcare providers or the patients.
- Affecting the trust and confidence of the patients and the public, such as by undermining the credibility, accountability, and transparency of the AI tools and the healthcare providers, or by violating the rights and dignity of the patients.
- Affecting the legal and ethical implications, such as by exposing the AI tools and the healthcare providers to potential lawsuits, penalties, or sanctions, or by violating the rules of healthcare.
Some of the possible solutions for transparency and explainability are:
- Improving the data documentation and annotation, such as by using metadata, labels, or tags, and making sure that the data sources, quality, and usage are traceable, verifiable, and auditable.
- Improving the algorithm documentation and validation, such as by using comments, diagrams, or metrics, and making sure that the algorithm logic, rationale, and criteria are understandable, interpretable, and testable.
- Improving the human feedback and communication, such as by using visualizations, explanations, or dialogues, and making sure that the human users, developers, and regulators can provide, receive, or exchange information, insights, or opinions about the AI tools and their processes and outcomes.
Privacy and Security
Privacy and security are the issues of ensuring that the personal and sensitive data and information of the patients and the healthcare providers are protected from unauthorized access, use, or disclosure. Privacy and security can be affected by various factors, such as:
- Data volume and variety, such as the use of large, diverse, or dynamic data sets, or the use of multiple, different, or distributed data sources, platforms, or devices, which may make the data and information more exposed or vulnerable.
- Data sharing and integration, such as the need to share, exchange, or integrate data and information among different AI tools, systems, or stakeholders, or the need to follow different rules, which may create conflicts or inconsistencies in the data and information governance or management.
- Data analysis and processing, such as the use of advanced, complex, or adaptive algorithms, or the use of cloud, edge, or decentralized computing, which may introduce risks or challenges in the data and information security or control.
Privacy and security can affect healthcare in many ways, such as:
- Affecting the quality and safety of care, such as by compromising the accuracy, completeness, or consistency of the data and information, or by causing errors, failures, or harms in the AI results or actions.
- Affecting the trust and confidence of the patients and the public, such as by undermining the confidentiality, integrity, or availability of the data and information, or by violating the rights and dignity of the patients.
- Affecting the legal and ethical implications, such as by exposing the AI tools and the healthcare providers to potential lawsuits, penalties, or sanctions, or by violating the rules of healthcare.
Some of the possible solutions for privacy and security are:
- Improving the data encryption and anonymization, such as by using techniques to protect the data and information from unauthorized access, use, or disclosure.
- Improving the data consent and authorization, such as by using mechanisms to collect, share, or use the data and information with the consent and authorization of the patients and the healthcare providers.
- Improving the data monitoring and auditing, such as by using tools to track, record, or report the data and information for any anomalies, incidents, or breaches.
Ethics and Regulation
Ethics and regulation are the issues of ensuring that the AI tools and their processes and outcomes are aligned with the moral values, principles, and standards of the healthcare sector and the society at large. Ethics and regulation can be affected by various factors, such as:
- Ethical dilemmas and conflicts, such as the trade-offs, risks, or harms of using AI tools that may go against the moral values, principles, or standards of healthcare or society.
- Regulatory gaps and uncertainties, such as the lack of clear, consistent, or comprehensive rules for the AI tools in healthcare, or the confusion or variation of how the rules are interpreted or applied.
- Stakeholder involvement and participation, such as the lack of awareness, understanding, or engagement of the people who use, develop, or regulate the AI tools, or the lack of representation, consultation, or empowerment of the patients and the public.
Ethics and regulation can affect healthcare in many ways, such as:
- Affecting the quality and safety of care, such as by causing errors, mistakes, or harms in the AI results or actions, or by giving wrong, incomplete, or misleading information or recommendations to the healthcare providers or the patients.
- Affecting the trust and confidence of the patients and the public, such as by undermining the credibility, accountability, and transparency of the AI tools and the healthcare providers, or by violating the rights and dignity of the patients.
- Affecting the legal and ethical implications, such as by exposing the AI tools and the healthcare providers to potential lawsuits, penalties, or sanctions, or by violating the rules of healthcare.
Some of the possible solutions for ethics and regulation are:
- Improving the ethical design and evaluation, such as by using frameworks, methods, or tools to identify, assess, or mitigate the ethical issues of the AI tools, and making sure that the AI tools are ethical and moral.
- Improving the regulatory development and implementation, such as by creating, updating, or harmonizing the rules for the AI tools in healthcare, and making sure that the AI tools and the healthcare providers follow the rules.
- Improving the stakeholder engagement and empowerment, such as by raising the awareness, understanding, or involvement of the people who use, develop, or regulate the AI tools, and making sure that the patients and the public are involved and empowered.
Future of AI in Healthcare
Artificial intelligence (AI) is transforming the future of work including the healthcare industry in unprecedented ways. As AI technologies become more advanced and accessible, they offer new opportunities for improving healthcare delivery, research, and innovation. Some of the emerging trends and opportunities of AI in healthcare are:
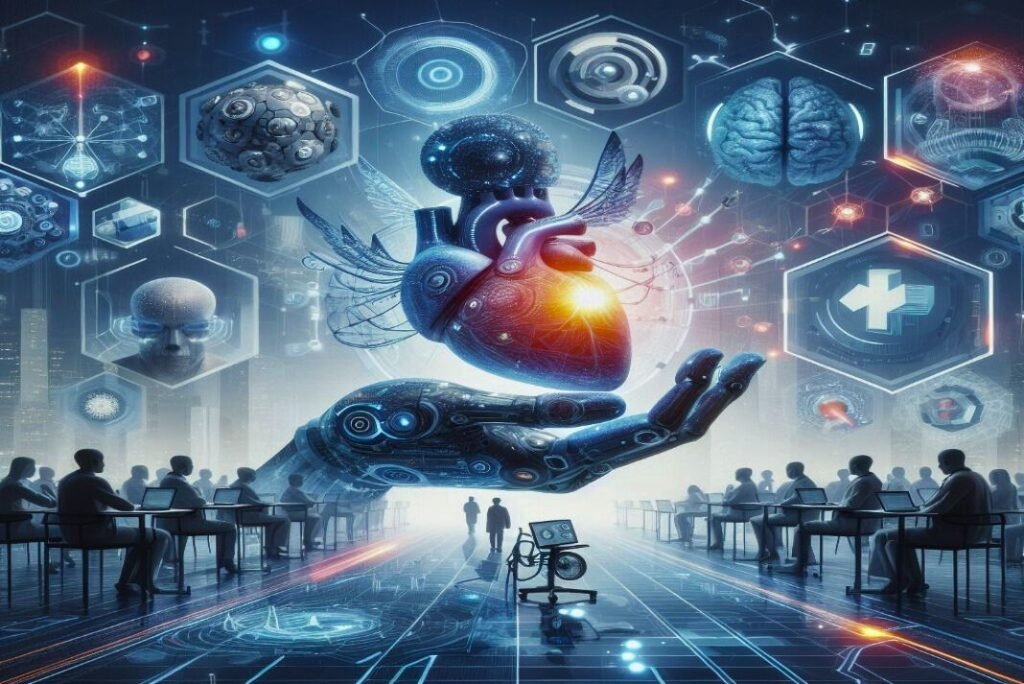
Emerging Trends and Opportunities
- Personalized medicine: AI can help tailor medical treatments to the individual characteristics and needs of each patient, such as their genetic profile, medical history, lifestyle, and preferences. AI can also help identify the optimal dosage, timing, and combination of drugs for each patient, as well as predict the potential side effects and interactions. Personalized medicine can improve the effectiveness and safety of treatments, as well as reduce the waste and cost of ineffective or harmful therapies.
- Precision public health: AI can help analyze large-scale data from various sources, such as electronic health records, social media, environmental sensors, and genomic databases, to identify patterns and trends in population health and disease transmission. AI can also help design and evaluate public health interventions, such as vaccination campaigns, disease surveillance, and outbreak response. Precision public health can help prevent and control epidemics, as well as address the social and environmental determinants of health.
- Digital therapeutics: AI can help deliver evidence-based interventions through digital platforms, such as mobile apps, wearable devices, and online programs, to prevent, manage, or treat various health conditions. AI can also help monitor and support the adherence and engagement of patients and providers with these interventions. Digital therapeutics can complement or substitute conventional therapies, as well as improve the accessibility and affordability of healthcare services.
- Augmented and virtual reality: AI can help create immersive and interactive simulations of real or imagined environments, such as operating rooms, patient scenarios, or anatomy models, using augmented reality (AR) or virtual reality (VR) technologies. AI can also help enhance the realism and feedback of these simulations, as well as adapt them to the needs and preferences of the users. Augmented and virtual reality can help improve the education and training of healthcare professionals, as well as the diagnosis and treatment of patients.
Skills and Competencies
As AI becomes more prevalent and influential in healthcare, it also poses new challenges and demands for the skills and competencies of healthcare professionals. Some of the skills and competencies that healthcare professionals need to develop or enhance in the era of AI are:
- Data literacy: Healthcare professionals need to be able to understand, interpret, and communicate data and information generated by AI systems, such as diagnostic results, treatment recommendations, or risk predictions. They also need to be able to critically evaluate the quality, validity, and reliability of these data and information, as well as the ethical and legal implications of their use.
- AI literacy: Healthcare professionals need to be able to understand the basic principles, capabilities, and limitations of AI technologies, such as machine learning, natural language processing, or computer vision. They also need to be able to interact with and oversee AI systems, such as providing feedback, correcting errors, or resolving conflicts.
- Human skills: Healthcare professionals need to be able to maintain and enhance the human aspects of healthcare, such as empathy, compassion, trust, and collaboration, in the presence of AI systems. They also need to be able to balance the benefits and risks of AI with the values and preferences of patients, providers, and society.
Conclusion
AI is a powerful and promising technology that can revolutionize healthcare in many ways. AI can help improve the quality and efficiency of care, reduce costs and errors, enhance patient experience and outcomes, and increase access and equity. However, AI also poses significant challenges and risks, such as data access and quality, bias and fairness, scaling and integration, transparency and explainability, privacy and security, ethics and regulation. Therefore, healthcare professionals need to be prepared and equipped with the necessary skills and competencies to harness the potential of AI, while ensuring its safe and responsible use.
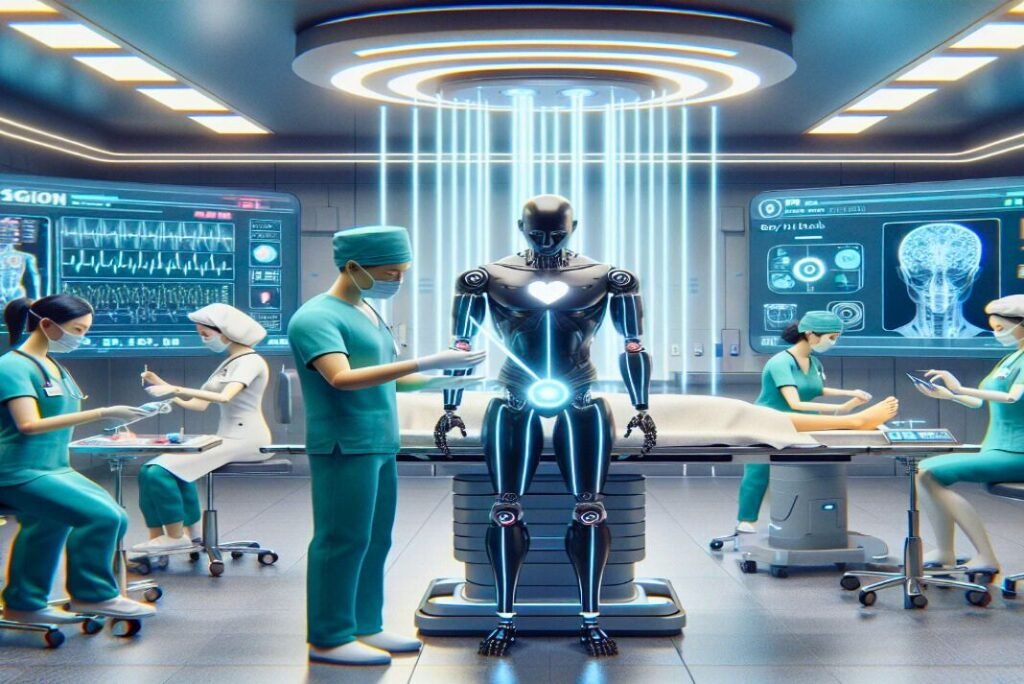
FAQs
Here are some frequently asked questions about AI in Healthcare: